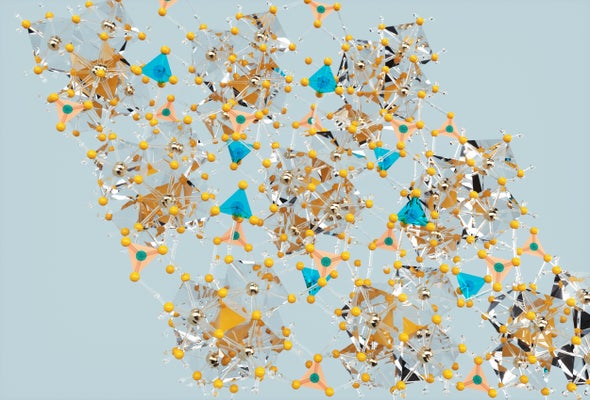
In my career as a chemist, I owe a huge debt to serendipity. In 2012, I was in the right place (IBM’s Almaden research lab in California) at the right time—and I did the “wrong” thing. I was supposed to be mixing three components in a beaker in the hope of systematically uncovering a combination of chemicals, meaning to replace one of the chemicals with a version that was derived from plastic waste, in an effort to increase the sustainability of thermoset polymers.
Instead, when I mixed two of the reagents together, a hard, white plastic substance formed in the beaker. It was so tough I had to smash the beaker to get it out. Furthermore, when it sat in dilute acid overnight, it reverted to its starting materials. Without meaning to, I had discovered a whole new family of recyclable thermoset polymers. Had I considered it a failed experiment, and not followed up, we would have never known what we had made. It was scientific serendipity at its best, in the noble tradition of Roy Plunkett, who invented Teflon by accident while working on the chemistry of coolant gases.
Today, I have a new goal: to reduce the need for serendipity in chemical discovery. Nature is posing some real challenges in the world, from the ongoing climate crisis to the wake-up call of COVID-19. These challenges are simply too big to rely on serendipity. Nature is complex and powerful, and we need to be able to accurately model it if we want to make the necessary scientific advances.
Specifically, we need to be able to understand the energetics of chemical reactions with a high level of confidence if we want to push the field of chemistry forward. This is not a new insight, but it is one that highlights a major constraint: accurately predicting the behavior of even simple molecules is beyond the capabilities of even the most powerful computers.
This is where quantum computing offers the possibility of major advances in the coming years. Modeling energetic reactions on classical computers requires approximations, since they can’t model the quantum behavior of electrons over a certain system size. Each approximation reduces the value of the model and increases the amount of lab work that chemists have to do to validate and guide the model. Quantum computing, however, is now at the point where it can begin to model the energetics and properties of small molecules such as lithium hydride, LiH—offering the possibility of models that will provide clearer pathways to discovery than we have now.
THE QUANTUM CHEMISTRY LEGACY
Of course, quantum chemistry as a field is nothing new. In the early 20th century, German chemists such as Walter Heitler and Fritz London showed the covalent bond could be understood using quantum mechanics. In the late the 20th century, the growth in computing power available to chemists meant it was practical to do some basic modeling on classical systems.
Even so, when I was getting my Ph.D. in the mid-2000s at Boston College, it was relatively rare that bench chemists had a working knowledge of the kind of chemical modeling that was available via computational approaches such as density functional theory (DFT). The disciplines (and skill sets involved) were orthogonal. Instead of exploring the insights of DFT, bench chemists stuck to systematic approaches combined with a hope for an educated but often lucky discovery. I was fortunate enough to work in the research group of Professor Amir Hoveyda, who was early to recognize the value of combining experimental research with theoretical research.
THE DISCONTENTS OF COARSE DATA
Today, theoretical research and modeling chemical reactions to understand experimental results is commonplace, as the theoretical discipline became more sophisticated and bench chemists gradually began to incorporate these models into their work. The output of the models provides a useful feedback loop for in-lab discovery. To take one example, the explosion of available chemical data from high throughput screening has allowed for the creation of well-developed chemical models. Industrial uses of these models include drug discovery and material experimentation.
The limiting factor of these models, however, is the need to simplify. At each stage of the simulation, you have to pick a certain area where you want to make your compromise on accuracy in order to stay within the bounds of what the computer can practically handle. In the terminology of the field, you are working with “coarse-grained” models—where you deliberately simplify the known elements of the reaction in order to prioritize accuracy in the areas you are investigating. Each simplification reduces the overall accuracy of your model and limits its usefulness in the pursuit of discovery. To put it bluntly, the coarser your data, the more labor intensive your lab work.
The quantum approach is different. At its purest, quantum computing lets you model nature as it is; no approximations. In the oft-quoted words of Richard Feynman, “Nature isn't classical, dammit, and if you want to make a simulation of nature, you'd better make it quantum mechanical.”
We’ve seen rapid advances in the power of quantum computers in recent years. IBM doubled its quantum volume not once but twice in 2020 and is on course to reach quantum volume of more than 1,000, compared with single-digit figures in 2016. Others in the industry have also made bold claims about the power and capabilities of their machines.
So far, we have extended the use of quantum computers to model energies related to the ground states and excited states of molecules. These types of calculations will lead us to be able to explore reaction energy landscapes and photo-reactive molecules. In addition, we’ve explored using them to model the dipole moment in small molecules, a step in the direction of understanding electronic distribution and polarizability of molecules, which can also tell us something about how they react.
Looking ahead, we’ve started laying the foundation for future modeling of chemical systems using quantum computers and have been exploring different types of calculations on different types of molecules soluble on a quantum computer today. For example, what happens when you have an unpaired electron in the system? Do the calculations lose fidelity, and how can we adjust the algorithm to get them to match the expected results? This type of work will enable us to someday look at radical species, which can be notoriously difficult to analyze in the lab or simulate classically.
To be sure, this work is all replicable on classical computers. Still, none of it would have been possible with the quantum technology that existed five years ago. The progress in recent years holds out the promise that quantum computing can serve as a powerful catalyst for chemical discovery in the near future.
QUANTUM MEETS CLASSICAL
I don’t envision a future where chemists simply plug algorithms into a quantum device and are given a clear set of data for immediate discovery in the lab. What is feasible—and may already be possible— would be incorporating quantum models as a step in the existing processes that currently rely on classical computers.
In this approach, we use classical methods for the computationally intensive part of a model. This could include an enzyme, a polymer chain or a metal surface. We then apply a quantum method to model distinct interactions—such as the chemistry in the enzyme pocket, explicit interactions between a solvent molecule and a polymer chain, or hydrogen bonding in a small molecule. We would still accept approximations in certain parts of the model but would achieve much greater accuracy in the most distinct parts of the reaction. We have already made important progress through studying the possibility of embedding quantum electronic structure calculation into a classically computed environment obtained at the Hartree-Fock (HF) or DFT level of theory.
The practical applications of advancing this approach are numerous and impactful. More rapid advances in the field of polymer chains could help address the problem of plastic pollution, which has grown more acute since China has cut its imports of recyclable material. The energy costs of domestic plastic recycling remain relatively high; if we can develop plastics that are easier to recycle, we could make a major dent in plastic waste. Beyond the field of plastics, the need for materials with lower carbon emissions is ever more pressing, and the ability to manufacture substances such as jet fuel and concrete with a smaller carbon footprint is crucial to reducing total global emissions.
MODELING THE FUTURE
The next generation of chemists emerging from grad schools across the world brings a level of data fluency that would have been unimaginable in the 2000s. But the constraints on this fluency are physical: classically built computers simply cannot handle the level of complexity of substances as commonplace as caffeine. In this dynamic, no amount of data fluency can obviate the need for serendipity: you will be working in a world where you need luck on your side to make important advances. The development of— and embrace of—quantum computers is therefore crucial to the future practice of chemists.
This is an opinion and analysis article.
"how" - Google News
March 15, 2021 at 09:01PM
https://ift.tt/2OYBP88
How Quantum Computing Could Remake Chemistry - Scientific American
"how" - Google News
https://ift.tt/2MfXd3I
https://ift.tt/3d8uZUG
Bagikan Berita Ini
0 Response to "How Quantum Computing Could Remake Chemistry - Scientific American"
Post a Comment