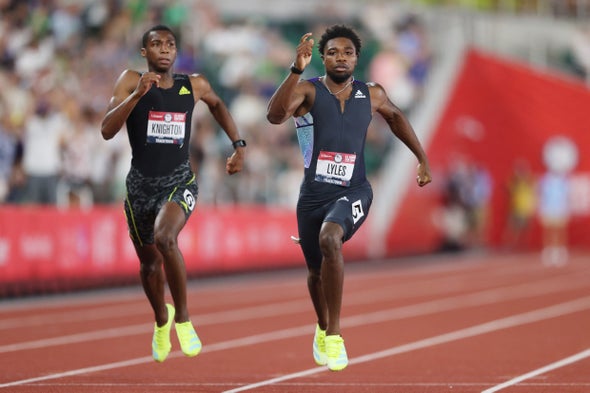
This year’s Olympic Games may be closed to most spectators because of COVID-19, but the eyes of the world are still on the athletes thanks to dozens of cameras recording every leap, dive and flip. Among all that broadcasting equipment, track-and-field competitors might notice five extra cameras—the first step in a detailed 3-D tracking system that supplies spectators with near-instantaneous insights into each step of a race or handoff of a baton.
And tracking is just the beginning. The technology on display in Tokyo suggests that the future of elite athletic training lies not merely in gathering data about the human body, but in using that data to create digital replicas of it. These avatars could one day run through hypothetical scenarios to help athletes decide which choices will produce the best outcomes.
The tracking system being used in Tokyo, an Intel product called 3DAT, feeds live footage into the cloud. There, an artificial intelligence program uses deep learning to analyze an athlete ’s movements and identifies key performance characteristics such as top speed and deceleration. The system shares that information with viewers by displaying slow-motion graphic representations of the action, highlighting key moments. The whole process, from capturing the footage to broadcasting the analysis, takes less than 30 seconds.
For example, during NBC ’s broadcast of the 100 meter trials in Eugene, Ore., the AI showed how Sha'Carri Richardson hit 24.1 miles per hour at her peak and slowed to 20.0 mph by the time she reached the finish line. That was enough to win the race: Richardson ’s runner-up hit a maximum speed of 23.2 miles per hour and slowed to 20.4 mph at the line.
“It ’s like having your own personal commentator point things out to you in the race,” says Jonathan Lee, director of sports performance technology in the Olympic technology group at Intel.
To train their Olympic AI via machine learning, Lee and his team had to capture as much footage of elite track and field athletes in motion as they could. They needed recordings of human bodies performing specific moves, but the preexisting footage used for similar research shows average people in motion, which would have confused the algorithm, Lee says. “People aren’t usually fully horizontal seven feet in the air,” he notes, but world-class high jumpers reach such heights regularly.
In the footage, a team at Intel manually annotated every part of the body—eyes, nose, shoulders, and more—pixel by pixel. Once those key points were identified, the model could begin connecting them in three dimensions until it had a simplified rendering of an athlete ’s form. Tracking this “skeleton” enables the program to perform 3-D pose estimation (a computer vision technique that tracks an object and tries to predict the changes it might undergo in space) on the athlete ’s body as it moves through an event.
The tracking system is limited to the track-and-field events at this year ’s games. But similar technology could become standard in a variety of sports, suggests Barbara Rita Barricelli, who is a human-computer interaction researcher and assistant professor at Italy ’s University of Brescia and is not involved with the Intel project. “The real big shift is when a technology is not only used for entertainment or research, but is accepted by the community of practice,” Barricelli says. For example, when video-assistant referees were first used in soccer, they were popular with broadcast networks—but some human referees refused to rely on them for game-changing decisions. The technology remains controversial, but now many officials routinely use the video assistant to help make a call. Baricelli suggests 3DAT ’s Olympic debut may be “a big step for research meeting practice—or better, practice embracing research results.”
Lee thinks the AI could help everyone from Olympians to average gymgoers correct their form, track changes in their gait that may indicate imminent injury, and more. “Long-term, what this technology will do is help improve [an] athlete’s performance by giving them more information,” two-time Olympic decathlon champion Ashton Eaton, who works for Intel on the 3DAT project, told the Oregonian.
All of this is only possible thanks to advances in computing that enable artificial intelligence to more effectively transform 2-D images into 3-D models. It ’s yielding “information we’ve never had before—that no one ’s ever had before—because it was too cumbersome,” Lee says. He thinks insights like those shared in the recent track-and-field trials are just the beginning.
In the future athletes will likely rely ever more on reams of data, processed with artificial intelligence, to up their game. One such tool may be a kind of model called the digital twin—“a virtual representation of a you-fill-in-the-blank,” says John Vickers, principal technologist for the Space Technology Mission Directorate at NASA Headquarters.
These models exist as data in a computer program, so they can be viewed on a screen or in virtual reality, and run through simulations of real-world situations. Vickers coined the phrase “digital twin” with Michael Grieves, a research professor at the Florida Institute of Technology, more than a decade ago. Vickers says engineers initially defined digital twins as constantly evolving virtual models of industrial objects, from the next generation of space-bound vehicles to entire Earthly cities. For example, in 2020 the U.S. Air Force began a six-year project to develop a digital twin of a B-1B Lancer bomber to understand how individual parts decay, and how to slow those processes. Now researchers are developing digital twins to build, test and even operate just about anything, ranging from abstract concepts like “fan experience” in an arena—to human beings.
Barricelli currently is working on exactly that. She believes engineers will soon be using data collected from wearable fitness monitors and AI tracking tools to deploy digital twins of individual athletes. Coaches could use these to test how competition is influenced by a wide variety of behaviors, from sleep patterns to diet to stance on the field. The twin could eventually help athletes make predictions about their future real-world performance, and could even suggest training adjustments.
“At that level, it would be really helpful for [athletes] to have continuous monitoring of the hypothetical outcome of their training,” Barricelli says. That way, “you see every time you do something how that affects the results you achieve.”
"how" - Google News
July 27, 2021 at 07:00PM
https://ift.tt/2UKh6IN
How Olympic Tracking Systems Capture Athletic Performances - Scientific American
"how" - Google News
https://ift.tt/2MfXd3I
https://ift.tt/3d8uZUG
Bagikan Berita Ini
0 Response to "How Olympic Tracking Systems Capture Athletic Performances - Scientific American"
Post a Comment